

Because the level of low-end compression will be different between the channel value and arcsinh transformed data, you may have different levels of background signal in each channel which will effect the FlowSOM grid and clustering results.Ģ. However, if there are serious structural differences between the R and FlowJo runs with FlowSOM, there could be a few causes:ġ. T cells in FlowJo were metacluster 1, but metacluster 5 in R, etc).
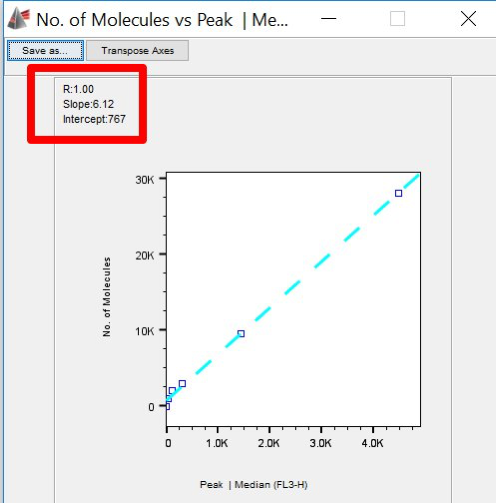
It's possible that the actual cell groupings could be comparable, just with different labels (e.g. one is 0 - 1024, and one is ~0 - 5), and the stochastic nature of clustering runs, mean the the cluster assignments might look quite different. In terms of the differences between your FlowSOM results from FlowJo and R, are these differences in the fundamental structure of the results, or just different cluster ID numbers of different groups of cells? The later is quite common and would be expected - as you have said, the simple fact that the values are going to be very different between the two datasets (e.g. The binning is done regardless of whether samples are combined or not - as the actual data doesn't play a role in determining the binning, only the plotted range does that. The helpful thing, is that if you have already optimised the axis settings for each channel (especially the extent of compression of the low-end values), then that adjustment is captured in the channel values for each marker individually. tSNE etc in FlowJo, or for when exporting data as CSV channel values). It is performed on all markers that are chosen for whatever function is being used (e.g. for CyTOF data, I think defaults would be something like -10^1 to 2x10^4 or, close to it). The range, in this case, is simply the minimum to maximum values that are _plotted_ by FlowJo (e.g. It is indeed the *range* that is split into 1024 uniformly ranged bins (as opposed to the bins containing equivalent numbers of cells). I'd like to implement the binning to see if my results are more similar to my collaborator's. The cluster expression patterns are fairly similar between my cluster mean heatmap and hers, but there are some differences that make me wonder if it's not just a matter of scaling and a different random seed. I have been running it on the biexponential transformed data, because I didn't know about the 1024-channel binning process until now. I am trying to reconcile the differences that I am seeing between a FlowSOM analysis that I have run in R with a FlowSOM analysis that my collaborator ran in FlowJo, on the same dataset. Is the binning done after combining data from different samples? And is it done within each marker or across all markers? Is the *range* of the data split into 1024 uniformly ranged bins, or are the actual data *values* split into 1024 bins with equal number of values in each bin? The former would be essentially a scaling with discretization while the latter would be transforming the data to ranks. I wonder if you have more detail about the binning algorithm. Thanks to tomash and Ian for your replies here. We have a page explaining some of this here. You can also export the data in this format (CSV channel values) if you want to use this for other clustering tools, rather than performing an arcsinh transformation - while the channel values do lose some information, it doesn't seem to really impact anything from my experience. With CyTOF it's not as important, as the channels will behave fairly similarly, but in flow data this might vary wildly depending on the level of spread. The benefit of this approach within FlowJo is that you can set up the level of low-end compression exactly as your data needs. When the tSNE/UMAP/whatever results are generated on the basis of these channel values, they are then attached to the _original_ data, so you never see the channel values. with a bi-exponential/logicle transformation etc) is turned into a linear set of data by binning the data into 1024 'bins' (in FJ10), reaching from the near side of the plot to the far side. Essentially the exact way you are plotting/visualising the data (i.e. To expand on Ian's helpful comments a little - FlowJo utilises the 'channel values' of the data.
